Lei Feng network news, Beijing time on June 8, Stanford University official website released a new achievement of Wu Enda team: radiologists use artificial intelligence algorithms to improve the diagnosis of cerebral aneurysms - cerebral aneurysms are bulges in the blood vessels of the brain May leak or rupture and may cause a stroke, brain damage or death.
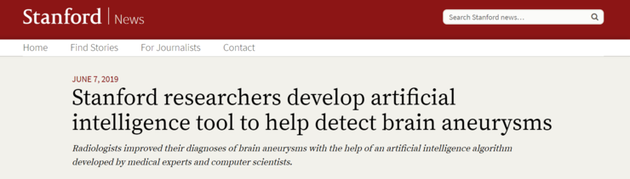
This achievement was published on the JAMA Network Open. Allison Park, a graduate student in statistics at Stanford University and co-author of the paper, said, "People have a lot of concerns about the practical role of machine learning in medicine. This study shows how humans can participate in the diagnostic process with the help of artificial intelligence tools. â€
According to Lei Feng.com, the tool is built around an algorithm called HeadXNet, which improves the ability of clinicians to correctly identify aneurysms at levels equivalent to the discovery of six additional aneurysms in 100 scans containing an aneurysm. In addition, it can increase the consensus of clinical interpreters.
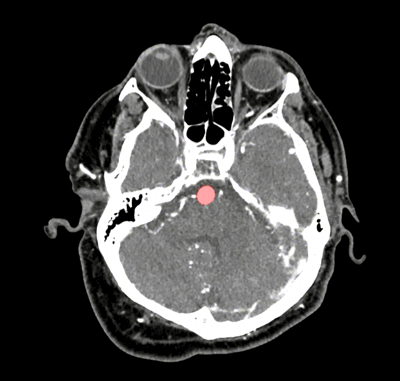
In brain scans, HeadXNet uses transparent red highlights to indicate the location of the aneurysm. (Source: Allison Park)
Although HeadXNet's success in these experiments is valuable, the research team cautioned that further investigation is needed to assess the robustness of AI tools prior to actual clinical deployment, as different hospitals have different device hardware and imaging protocols to study People plan to solve these problems through multi-center cooperation.
Physicians reduced the rate of missed diagnosis with the help of AI
Carding brain scans and finding aneurysms means browsing hundreds of images. Aneurysms come in a variety of sizes and shapes and expand outward at different angles - some aneurysms are just a spot in a series of movie-like images.
“Finding an aneurysm is one of the most laborious and critical tasks for radiologists,†said Kristen Yeom, associate professor of radiology and co-author of the paper. “Considering the inherent challenges of complex neurovascular anatomy, and The fatal consequences of missing aneurysms prompted me to apply the advances in computer science and vision to neuroimaging."
Yeom brought this idea to the AI ​​for Healthcare Bootcamp, run by the Stanford Machine Learning Group, led by Associate Professor of Computer Science and co-author of the paper, Andrew Ng. The core challenge of the team was to create an artificial intelligence tool that could accurately process these large numbers of 3D images and complement clinical diagnostic practices.
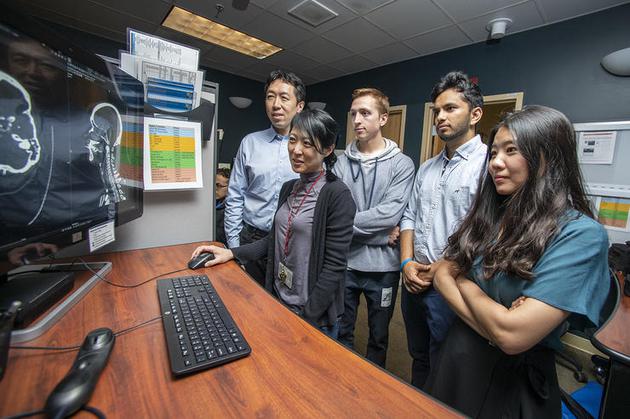
HeadXNet team members from left to right are: Andrew Ng, Kristen Yeom, Christopher Chute, Pranav Rajpurkar and Allison Park (Source: LA Cicero)
To train their algorithms, Yeom teamed up with Park and computer science student Christopher Chute to collect 611 clinically significant aneurysms detected in head CT angiography.
"We manually tagged each voxel - the equivalent of a 3D image of a pixel - whether it is part of an aneurysm," Chute said. "Building training data is a daunting task with a lot of data."
After training, the algorithm determines if each voxel scanned has an aneurysm.
The end result of the HeadXNet tool is that the algorithm's conclusion is highlighted at the top of the scan with a translucent highlight. This representation of algorithmic decisions allows clinicians to easily see scan results without HeadXNet input.
“What we are interested in is how these scans with artificial intelligence will improve the performance of clinicians,†said Pranav Rajpurkar, a computer science student and co-leader of the paper. “We can mark the exact location of the aneurysm to the clinician, not just the algorithm that says the image contains an aneurysm.â€
By evaluating a brain scan of a group of 115 aneurysms, eight clinicians tested HeadXNet, one with the help of HeadXNet, and one at a time.
With this tool, the clinician correctly identified more aneurysms, which reduced the “missing rate†and the doctors were more likely to agree. In addition, HeadXNet does not affect the time required for the clinician to determine the diagnosis, nor does it affect the ability of the physician to correctly identify the scan if the patient does not have an aneurysm.
Not just the automation of artificial intelligence
Lei Feng.com understands that the core machine learning method of HeadXNet may be used to identify other diseases inside and outside the brain. For example, Yeom envisions future versions that can focus on accelerating the identification of aneurysm ruptures, saving valuable time in an emergency. However, there are still significant barriers to integrating any artificial intelligence medical tools with the daily clinical workflow of the hospital radiology department.
The current scan viewer is not designed to work with deep learning, so researchers have to develop custom tools to integrate HeadXNet into the scan viewer.
Similarly, changes in real data—as opposed to data tested and trained by the algorithm—may reduce model performance. If the algorithm processes data from different kinds of devices or imaging protocols, or processes data that is not part of its original trained patient population, then it may not work as expected.
Wu Enda said: "Because of these problems, I think the deployment speed will be faster, not just artificial intelligence automation, but the cooperation between artificial intelligence and radiologists. We still have technical and non-technical work to do, but as a team, We will achieve this goal, and the cooperation between artificial intelligence and radiologists is the most promising approach."
Source: Lei Feng Net
Laminated Fabric,Two Layer Lamination,Multi Layer Lamination,Laminated Printing Non Woven Fabric
ShaoXing SurgeCare Medical Products Co., Ltd. , https://www.sxsurgecaremedical.com